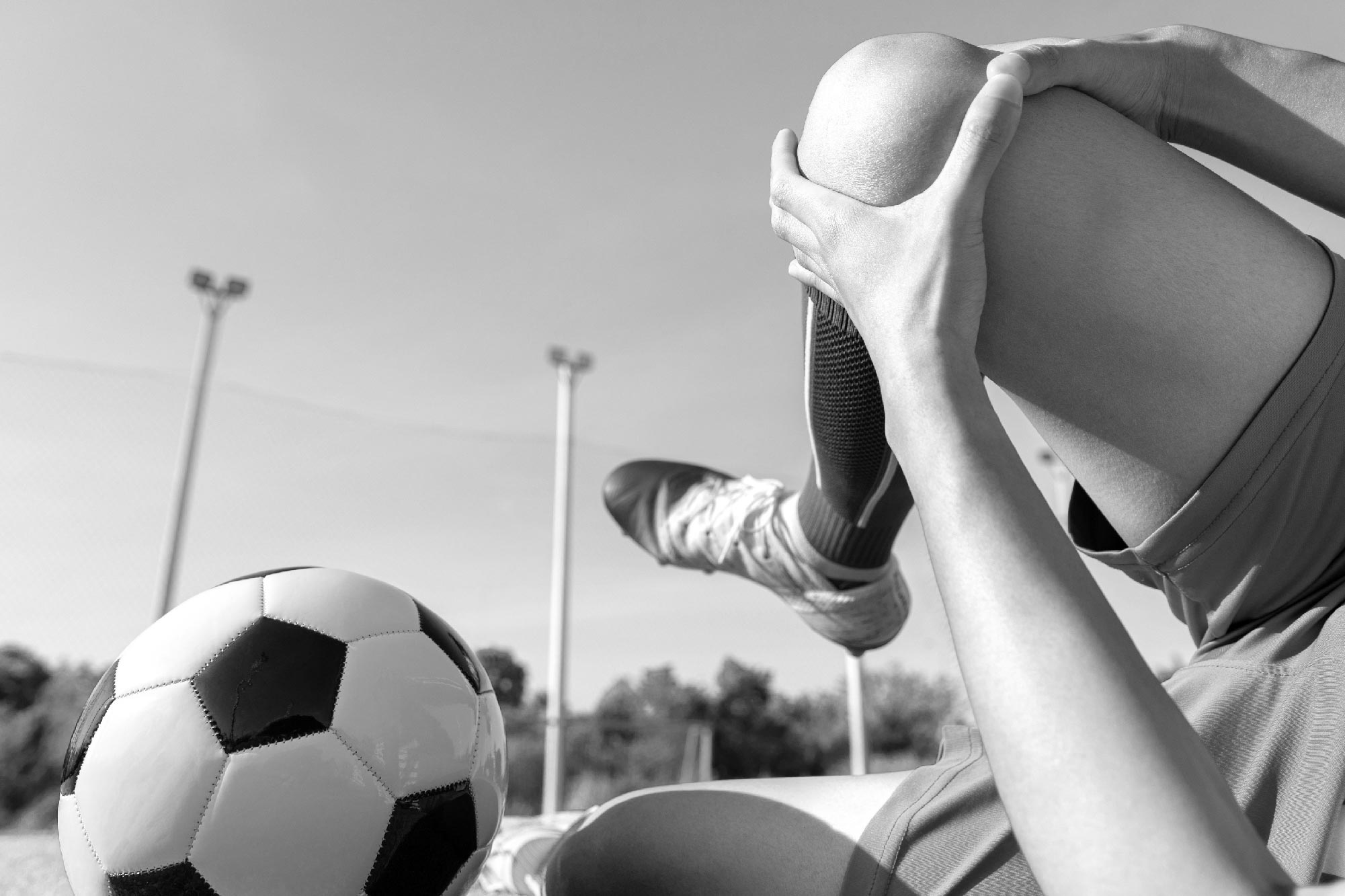
CLIENT’S NEED
In March 2020, the World Health Organization declared the outbreak of COVID-19 originating in Wuhan, China, to be a pandemic. The containment measures – including social distancing and confinement – halted most economic activities.
Among the stopped activities was the practice of most competitive sports. Never in history have athletes had such a long forced collective break. The only precedent in elite sports occurred in the United States in the NFL (American Football). During 4 months in 2011, the sports competition was stopped due to a strike by the athletes. In the case of the Spanish Professional Football League (LFP), after a three-month hiatus (both the competition and the training of all the athletes), the season resumed 11 days before the end of La Liga.
Given their extensive experience and track record, Ripoll and De Prado Sports Clinic already anticipated an increase in the number of injuries when competition resumed. However, it was necessary to quantify the extent of the potential harm caused by this break in order to recommend training and injury prevention guidelines. Recognizing their limitations in math and A.I, they contacted Biyectiva for a numerical prediction of the number of injuries.
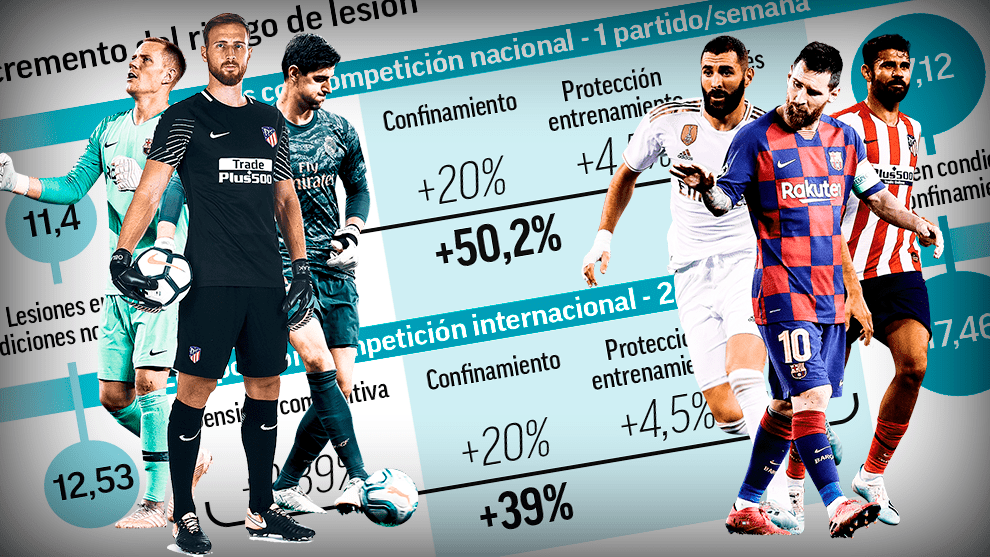
CHALLENGES AND DIFFICULTIES
The only precedent for which reliable historical data is available refers to American football, a sport with a prevalence and type of injury very different from football. Elite competition teams are very wary of publicly sharing data on player injury and therefore data is scarce; a crucial factor in the design of Artificial Intelligence systems.
Another difficulty encountered is related to the scientific articles published on the subject that exceed the simplification of reality and often omit the effect of lack of training on soccer players (given that a break of such length is unheard of). Therefore, there were no previous references in the design of mathematical models for this purpose.
SOLUTIONS
After an in-depth study of the scientific literature, as well as the probabilistic injury estimation techniques and models, a screening and separation of variables was carried out: quantitative based on existing data, qualitative indeterminate (due to lack of precedents) and qualitative quantifiable (within a few margins).
Thanks to a series of precise historical data that UEFA collects on base injury rates in different teams in European competitions, a mathematical regression model was developed. The goal was to be able to estimate how a two month break in training, in addition to the intensive training load upon return, would affect injuries in the future.
On this mathematical model, the various factors outlined by the scientific literature were incorporated and, in this way, a global predictive model was created for the quantification of qualitative variables.
RESULTS
The return to the playing fields determined the beginning of the evaluation of the injury model created by Biyectiva. Two different periods were factored in during the course of the remaining days: approximately halfway through the competition as well as at the end of it. Both evaluations demonstrated the high accuracy of the predictive model, with rates of 95.7% and 99%. As a curious fact, our prediction for the end of the season was that there would be 40.4 injured players and, in reality, it turned out to be 40.
Thanks to the great precision of the model created by Biyectiva, Ripoll and De Prado Sports Clinic were able to reaffirm the importance of sports-medical preparation in the prevention and forecasting of injuries. You can read about the development and results of this project in the three articles published in the Marca newspaper.
The risks of the 'new' football in the pandemic
Football The 'COVID factor': Muscle injuries will mark the season
Post-Covid football brought 116 injuries and an increase of 45%
After the success of the injury model and with the knowledge acquired, the Biyectiva team also developed, together with Ripoll and De Prado's team, an algorithm to estimate a player's injury rate based on his own history. A very personalized estimation of each player taking into account his history of injuries, physical condition, the position he occupies on the field, and other biometric data of the player.
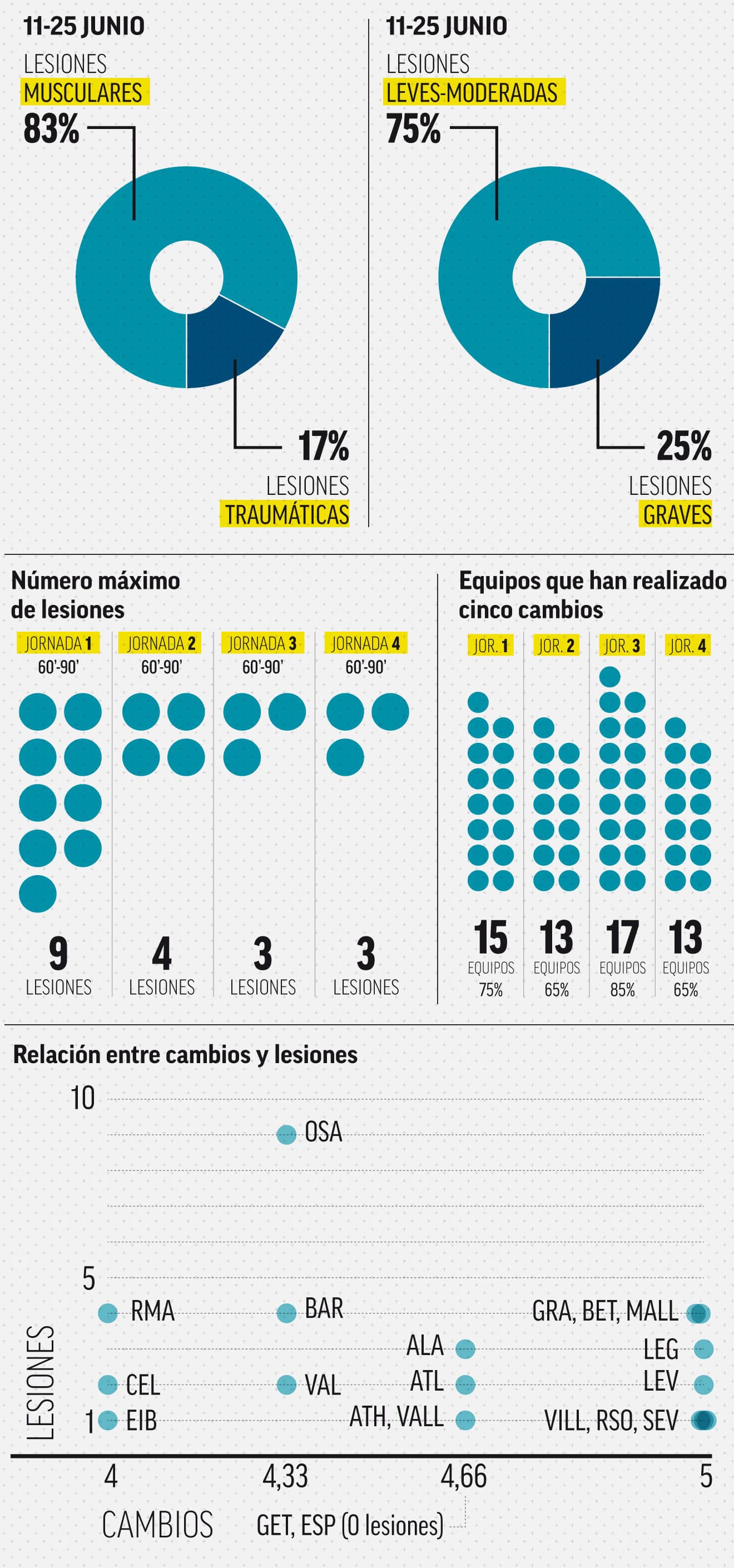
Font: marca.com